The quandary of health data in the AI era: privacy or fairness?
On June 20 2024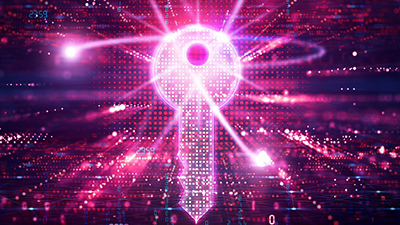
Updated on February 13, 2025, to reflect the latest information.
This article was republished from The Conversation (FR) by Nesrine Kâaniche, associate professor, cybersecurity and privacy expert, member of the VP-IP IMT Chair and Aymen Boudguiga, cybersecurity research engineer at the French Alternative Energies and Atomic Energy Commission (CEA).
Artificial intelligence (AI) is spreading to all areas of our daily lives as it revolutionizes areas such as medical diagnostics, transportation, finance and security. It is increasingly affecting our decision-making by collecting and analyzing large quantities of our data that drive decision-making algorithms and influence our choices. This pervasiveness of AI raises important ethical and social questions along with a complex issue with no easy answer: should we trust it?
The EQUIHid project explores the potential of federated learning to make health services fairer and privacy friendly. Federated learning seeks to train artificial intelligence models collaboratively. It is used to address a specific problem, such as the analysis of mammographic images for early detection of cancer and identification melanoma in images of skin lesions. The model is trained on large amounts of patient data from different hospitals.
AI models reproducing inequalities
Imagine a model designed to diagnose skin cancer that can detect cancer with remarkable accuracy in patients with light skin but is drastically less effective when it comes to darker skin. The model is considered to be unfair because it unjustly favors one group of patients over another. How does this happen?
The answer lies in the data used to train this model. If the data is biased due to an overrepresentation of a certain patient type, the model will perform better with this group, which produces the bias observed in the data.
FIND ANALYSIS, NOT INVECTIVE ON THE CONVERSATION.
If the training database primarily contains images of light-skinned patients, the model will be less exposed to pigmentation variations and forms of cancer that occur more frequently in dark skin. It will therefore be less effective in diagnosing melanoma in patients with dark skin tone. This kind of bias can have serious consequences. A misdiagnosis or delayed diagnosis can negatively affect a patient’s prognosis. Moreover, these biases reinforce existing inequalities in the health system, creating a disadvantage for groups that are already marginalized.
This type of bias increases when the large volumes of data used to train these models are not representative of the general population. The medical data used for training comes from hospital consultations. However, since each institution has only a partial view of the problem, based on its local population, it will face challenges in obtaining a fair model. One solution to this problem is to enhance the data by combining different data sources to ensure fair federated learning.
Fairness, privacy and decentralized learning
How does this work? Several entities communicate with each other directly to enable cooperation without sharing any potentially sensitive data and without centralizing the data in a common site managed by a third party. While this ensures data sovereignty, it is not enough to guarantee patients' privacy in the learning bases. Although their data is not directly exposed, it is used to train models which can reveal sensitive health information in the event of a cyber-attack.
Let’s look again at the example of a model for skin cancer diagnosis. An attacker could interact with the model to try to guess personal details about a particular patient, including the patient’s likelihood of developing the disease. If the model responds with great certainty, this indicates that the patient in question was probably present in the training set, which reveals their predisposition to this disease, without direct access to their medical data.
The EQUIHid project aims to design new federated learning algorithms that simultaneously ensure privacy and decentralize the training of non-discriminatory models. In addition to algorithmic developments, one unique characteristic of the project is the aim of studying the problem theoretically in order to assess how fairness impacts the models’ performance. How does fairness interact with federated learning? How does it interfere with privacy-friendly learning? Finally, how do the three concepts interact together?
Although the areas of equity, privacy and federated learning have been extensively studied individually, their interactions are rarely considered in the current scientific literature. It is therefore important to find the right balance to solve this three-parameter equation.
A move towards the implementation of fairer models
Researchers at the National University of Singapore (NUS) have shown that fairness in machine learning models comes at a cost in terms of privacy. Furthermore, this cost is not evenly distributed: the leak of information linked to learning models is significantly greater for disadvantaged sub-groups, who are precisely the ones for whom fair learning is so crucial. Through EQUIHid, we demonstrated that the more biased the training data, the higher the privacy cost for achieving fairness for these subgroups will be.
During the first phase of the project, we explored an existing solution, FairFed, which uses several models which are more or less fair to build a comprehensive model that is fairer with than the ones it aggregates. We sought to extend this approach to cover additional constraints, including privacy. To accomplish this, we introduced a first proposal based on homomorphic encryption and differential confidentiality techniques.
Homomorphic encryption is a cryptographic technique that allows you to perform mathematical operations on encrypted data, without having to decrypt it first. This ensures the confidentiality of the data during processing. Differential confidentiality, on the other hand, is a mathematical property of statistical data that ensures that it is very difficult to infer whether or not a specific individual is present in a data set, even after aggregated statistics are published.
Human-centered AI
Our solution is founded on these concepts and can be used to train a single model based on several encrypted models from different entities. As it aggregates them, it also weighs their participation according to their level of fairness. This ensures greater confidentiality for the training data and develops a fairer aggregate model.
During the second phase of the project, we will address the issue of the integrity of federated learning to ensure the model training runs smoothly and to avoid any deviations which could have significant consequences, such as the generation of a biased model leading to erroneous medical diagnoses or the massive leak of sensitive data.
The issue of AI and fairness has become a priority for European and international institutions. The Artificial Intelligence Act (AI Act), adopted by the European Parliament in March 2024, highlights the importance of ensuring the fundamental rights of data protection, human dignity and non-discrimination. Research aimed at detecting, reducing and even eliminating biases in learning models is therefore essential in promoting fairer, human-centered AI.
The EQUIHid project is supported by the French National Research Agency (ANR), which funds project research in France. Its mission is to support and promote the development of fundamental and finalized research in all disciplines, and to foster dialogue between science and society. Visit the ANR website for more information.
⇒ This article was republished from The Conversation under the Creative Commons license. Read the original article (fr).